PhD Student: Rachel Field; Partner: Helios; Supervisors: Prof. Jennifer Cook and Dr. Connor Keiting; School: School of Psychology.
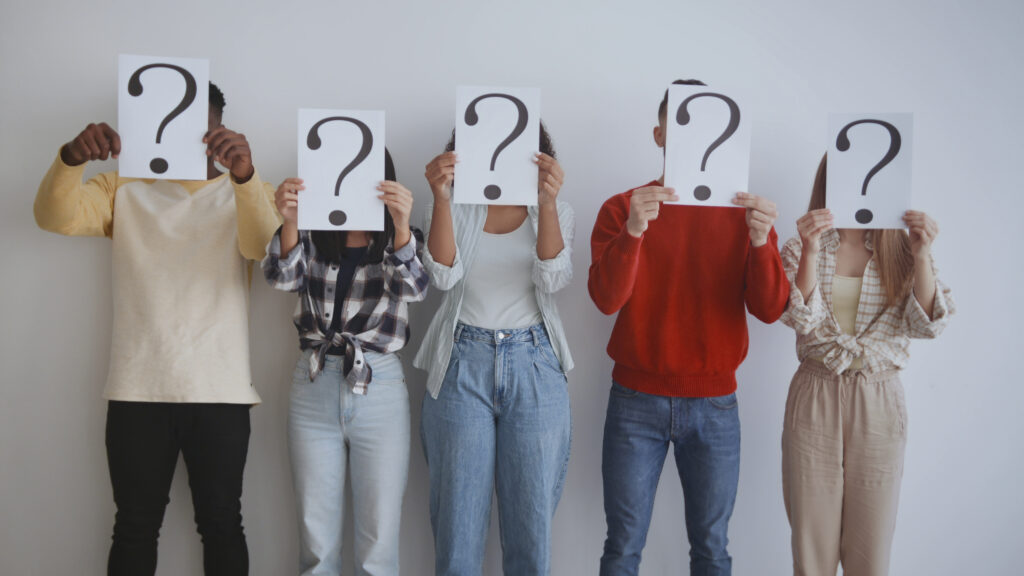
Currently, access to autism diagnosis in the UK is subject to delay and inequity. Increasing numbers of referrals to autism assessment services mean that autistic children, especially autistic girls and children of colour, and their families are often waiting unacceptable lengths of time to access essential early support. This is in part due to ineffective screening tools. Current tools are rife with inaccuracy and bias, often leading to over-identification of autism whilst also missing those from racial and gender minorities. This project aims to address these issues through the development of objective, digital tools to facilitate timely and unbiased autism screening.
Previous studies have shown that movement analysis can be an effective way to distinguish autistic and non-autistic samples. Recent pioneering research has seen the creation of tools which successfully identify autism in adult samples across all genders and racial groups with high precision. This is done through algorithms which extract certain movement features from posed and spoken emotional facial expressions (FaceMap) and tracing the outlines of shapes (ShapeMap). In order for these tools to facilitate early autism screening, the next step is to adapt them for autistic and non-autistic children, and then assess their efficacy for distinguishing these groups.
Therefore, this project will adapt these current tools to produce child-friendly smartphone games which can record facial and bodily movement data through video recordings and drawing games. Through interviews and focus groups with autistic and non-autistic children and their caregivers (Study 1), we can then test and improve the accessibility of these tools to ensure they can be administered without researcher involvement, which will be key for any future implementation of these tools in autism assessment services. Following this, we will collect data from both autistic and non-autistic children to characterise and compare their facial (Study 2) and bodily (Study 3) movements. Using machine learning techniques, we will create a classifier tool to distinguish between autistic and non-autistic forms of movement, and assess the efficacy of this tool, particularly for autistic girls and children of colour (Study 4).
The anticipated outcome of this project is the creation of a tool which can be used to improve screening for autism and facilitate equitable access to diagnosis across demographic groups, thereby improving long-term outcomes for autistic people and their families through access to earlier support.